5 September 2024
Industry Insights
Why we need new standards to measure AI energy efficiency
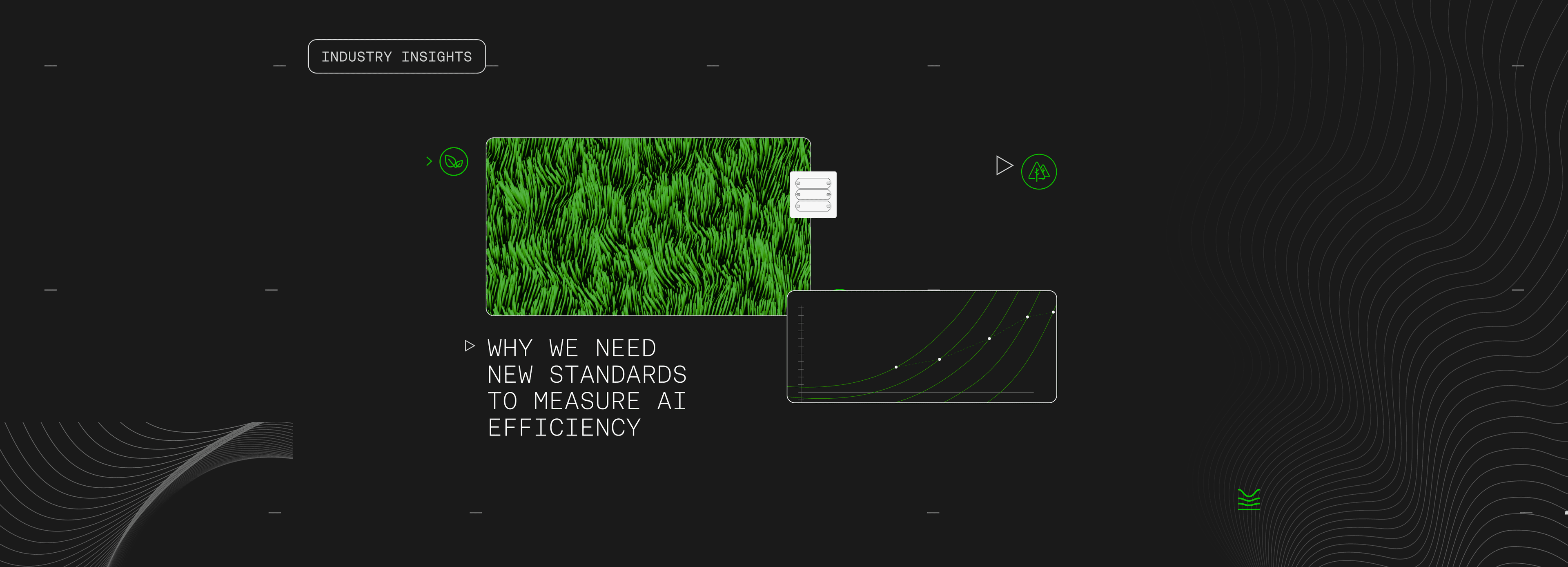
For the longest time the data centre industry has relied on power usage effectiveness (PUE) as a de facto metric to determine the energy efficiency of data centres. The PUE is calculated by dividing the total facility power with the power consumed by its IT equipment, offering a simple way for the industry to identify inefficient deployments and compare data centres.
Increasingly, government agencies seeking to raise the bar on sustainability have turned to PUE. In China, a joint plan released in July by the National Development and Reform Commission, the Ministry of Industry and Information Technology, and two central bureaus, called for the lowering of PUE to below 1.5 by 2025.
And in its Green Data Centre Roadmap released in May this year, the Singapore Infocomm Media Development Authority (IMDA) outlined its goal for all data centres in Singapore to achieve a PUE of less than or equal to 1.3 over the next decade.
The limitations of PUE
However, while PUE does have its uses, the established metric has well-known limitations. For a start, PUE is often measured under ideal conditions when the IT load is at its peak. This means it is unlikely to reflect typical operations, especially during periods of low demand or where workloads are dynamic.
PUE also doesn’t consider climate impact. Two data centres with the same PUE located in different parts of the world will have very different real-world efficiency that belies their comparable PUE ratings. Moreover, the impact of highly efficient cooling strategies such as direct-to-chip and immersion cooling are not adequately captured by PUE, as power-hungry fans installed in servers are classified as IT power.
“ This means appraising a facility solely using its PUE makes it prone to manipulation, gives a skewed view of the facility’s true efficiency, and offers only a limited, point-in-time measurement. Crucially, the PUE doesn’t measure the useful output of a data centre. ”
To address these shortcomings, we need more comprehensive metrics that take the actual workload, performance and energy sustainability into account. Specifically, we need to focus on the work done with a certain amount of energy.
A gauge for accelerated computing
The challenge of accurately determining energy efficiency is only getting more pronounced with each new generation of computer systems. Consider the latest microprocessors and GPUs, which consume more power than before, pushing the limits of traditional cooling solutions and data centre designs.
Yet if one looks beyond their energy consumption, one will find that they produce much more work for the amount of energy they use. Indeed, Stanford University’s Human-Centered AI group estimated (PDF) that GPU performance has increased some 7,000 times since 2003 with a 5,600-fold improvement in price per performance.
In May, Nvidia quoted Jonathan Koomey, a researcher and author on computer efficiency and sustainability, in a blog post. Koomey suggested using 'tokens per joule' to measure the implications of AI workloads and advocated for broader discussions to establish benchmarks in this area.
“Companies will need to engage in open discussions, share information on the nuances of their own workloads and experiments, and agree to realistic test procedures to ensure these metrics accurately characterise energy use for hardware running real-world applications,” said Koomey.
Start with energy consumption
In the meantime, is there a way to determine power efficiency at a more granular level than what PUE offers? Fortunately, this year’s MLPerf benchmarks not only added tests for two generative AI models but also invited contributions regarding energy consumption.
In the MLCommons-verified report released in July, SMC’s GPT-3 175B, 512 H100 Tensor Core GPUs submission consumed only 468 kWh of total energy when connected with NVIDIA Quantum-2 Infiniband networking. This demonstrates significant energy savings over conventional air-cooled infrastructure, which, when combined within our Singapore data centre, has proven to save nearly 50% total energy^.
This is possible because of our focus on an end-to-end strategy with our HyperCube since the very beginning. We pushed the limits of single-phase immersion cooling, designing every element to create a unique environment that lowers energy use by almost half.
This didn’t come at the cost of performance. Our systems achieved performance within a few per cent of the average for systems up to 64 nodes/512 H100 GPUs, delivering class-leading results in both GPU performance and energy consumption.
Only Sustainable Metal Cloud submitted power data for this benchmark. Peer-reviewed power data of equivalent standard cooled servers were used for comparison.
Moving forward
To truly advance in this area, the industry must embrace a paradigm shift towards metrics that adequately capture both the energy consumption and output of computer systems. As AI workloads grow in data centres, we need new standards to measure AI energy efficiency.
At SMC, our commitment to reducing energy consumption without compromising performance demonstrates that it is possible to achieve both environmental responsibility and technological excellence. This paves the way for a more sustainable future in technology.
As we continue to innovate and refine our technology, we remain dedicated to leading the industry towards greener practices, ensuring that our advancements positively contribute to the global effort in combating climate change.