13 June 2024
Updates
The world's first MLPerf® Training power consumption results
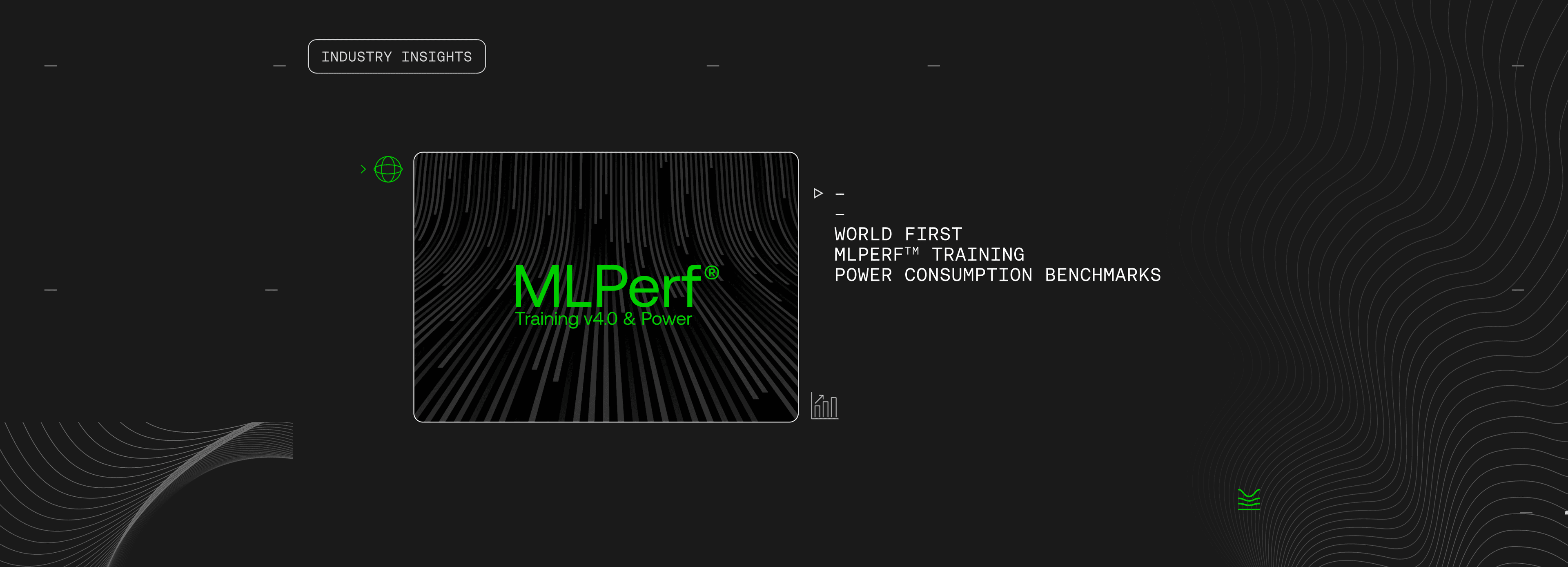
We’ve been hard at work at Sustainable Metal Cloud and are thrilled to share some groundbreaking news with you. As one of the newest members of MLCommons, we’ve just released our first MLPerf® Training benchmark results.
For the first time ever, submitters can publish the power data of their equipment during testing, and guess what? We’re the only member to publish a full suite of power results along with performance data for training on clusters as large as 64 nodes (512 GPUs).
“ MLPerf’s benchmarks exist to drive innovation in machine learning. It’s fantastic to see our newest member, Sustainable Metal Cloud, submit with our first-ever power measurements. This sets a new standard for energy efficiency in AI training. ”
We are committed to transparency. View all our MLPerf results via our Research page, including a power consumption calculator that breaks down energy and CO2 savings.
Our GPT-3 175B, 512 H100 Tensor Core GPUs submission consumed only 468 kWh of total energy when connected with NVIDIA Quantum-2 Infiniband networking. This showcases significant energy savings over conventional air-cooled infrastructure, which when combined within our Singapore data centre, has proven to save close to 50% total energy.
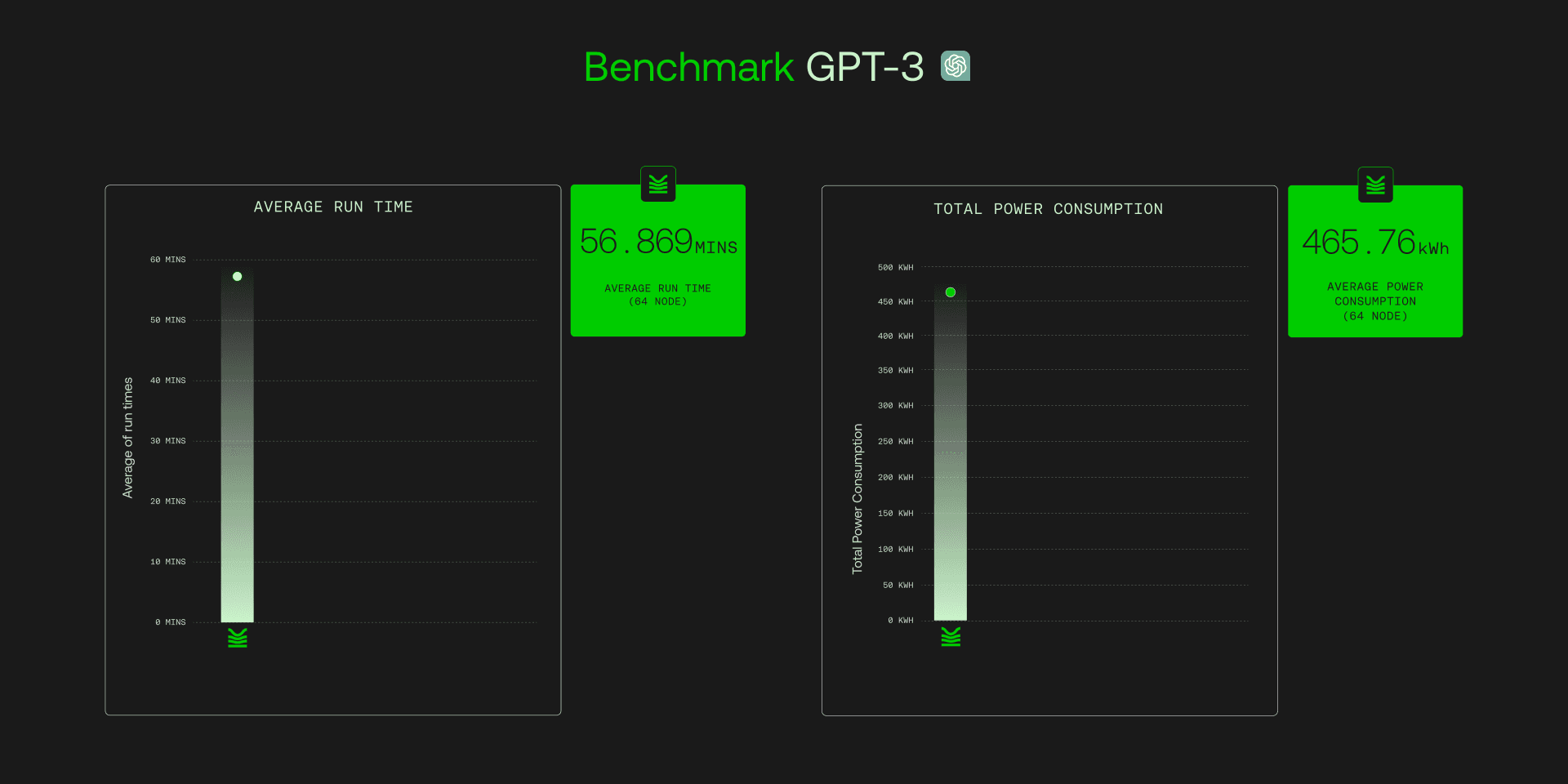
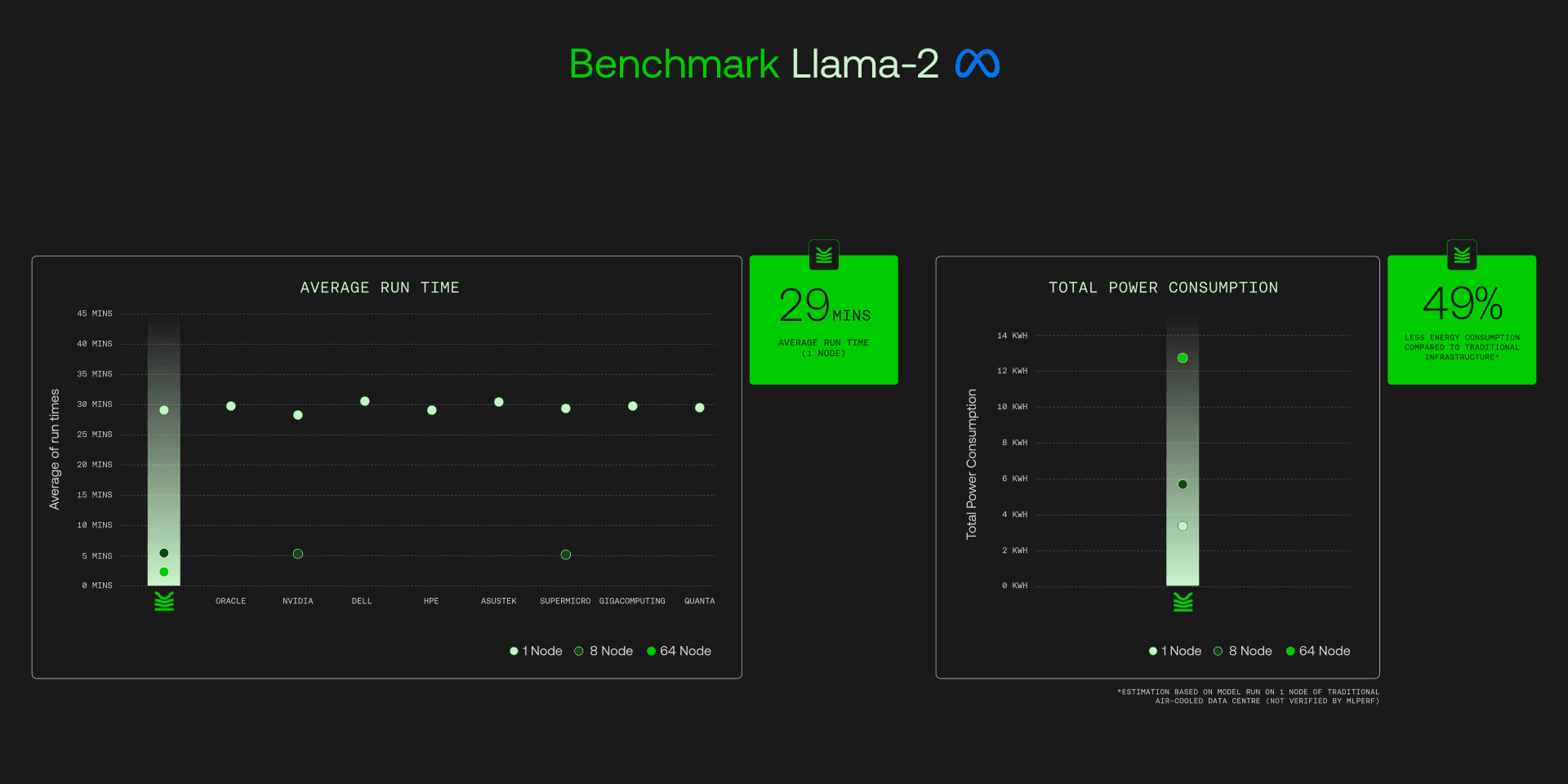
Furthermore, we are one of the world’s first clouds to enable VBOOST. By adopting an enhanced VBOOST-enabled software stack post-submission, we achieved further energy reductions to 451kWh and a 7% performance improvement, positioning OUR customer cloud training performance environment just 6% below NVIDIA’s flagship Eos AI supercomputer.
David Kanter further added, “Our benchmarks help buyers understand how systems perform on relevant workloads. The addition of the new power consumption benchmark gives both our members and buyers a new way to rate output efficiency, as well as environmental impact. Sustainable Metal Cloud’s release establishes a baseline for best practice power consumption. As a community, it’s important that we can measure things so we can improve them. I hope SMC’s initial results will help drive transparency around the power consumption of machine learning training.”
So, how did we get the same flops with half the energy? We’ve developed an AI GPU cloud using our proprietary single-phase immersion platform, known as “Sustainable AI Factories.” The magic happens in our Sustainable AI Factories. Around 30% of our energy savings come from the immersion hardware itself, while the remaining 20% comes from data centre-level savings.
“ We are thrilled to be a part of MLCommons and contribute to advancements in energy-efficient AI infrastructure. MLCommons has provided us with the platform to validate our technology which now presents as a viable solution to host the next generation of AI compute, using less power, water, and space. ”
So, what have we measured?
For each MLPerf® training result, we measured the power consumption of each 'node' or GPU server. This was captured as far upstream as possible - at the level of our immersion-rack power shelves - to assess each node's power consumption accurately. This portion of our MLPerf® submission was reviewed and verified by MLCommons and other members. At this level, our results show approximately 30% better performance compared to H100 SXM-based systems cooled with air, the most common method for building GPU clusters today.
However, capturing power at the node is only part of the story. The host data centre plays an incredibly important role in measuring the energy efficiency of an AI Factory. Data centre power usage was not within the scope of this round of MLPerf®, but we hope it will be included in future evaluations. Measured by PUE (Power Usage Effectiveness), our data centre level provides a (non-MLCommons-verified) estimate of the total power required for the relevant test - end to end.
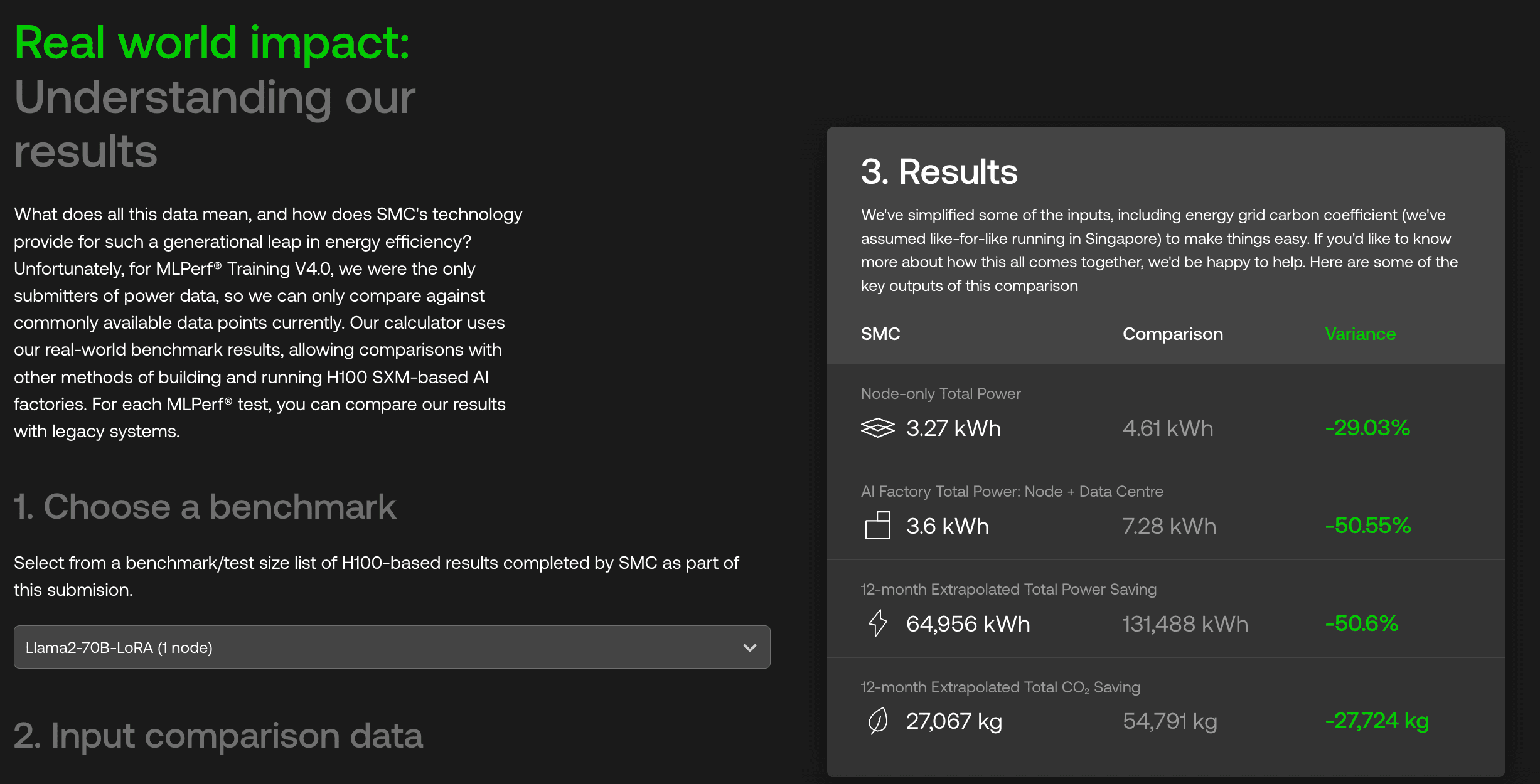
Our operations are primarily based in Asia, and we’re expanding our network globally with scaled GPU clusters and infrastructure, including the powerful NVIDIA H100 SXM accelerators.
We’re dedicated to pushing the envelope on energy efficiency in AI training, and our results, verified by MLCommons®, speak volumes about our commitment.
This is why we’re here at Sustainable Metal Cloud – to lead the way in energy-efficient AI. We hope our initial results will help drive transparency around the power consumption of machine learning training and set new benchmarks for the industry.
For media enquiries: lauren.crystal@smc.co
For partnership enquiries: tim.rosenfield@smc.co