Global leadership: Our MLPerf® Training & Power V4.0 results
Performance with efficiency: Energy use & our MLPerf® Training V4.0 results
Our mission is simple: to convert electrons into knowledge as efficiently as possible. We believe transparency - of all AI's cost inputs - is the only way to move the industry towards genuine efficiency. We’re moving the industry forward by releasing the world’s first MLPerf® certified training power consumption benchmarks.
Our power results are comprehensive. Factoring in our data center energy requirements, they provide the first open-book look at the end-to-end power requirements for building and running an AI Factory: how much energy goes in, and how much knowledge comes out.
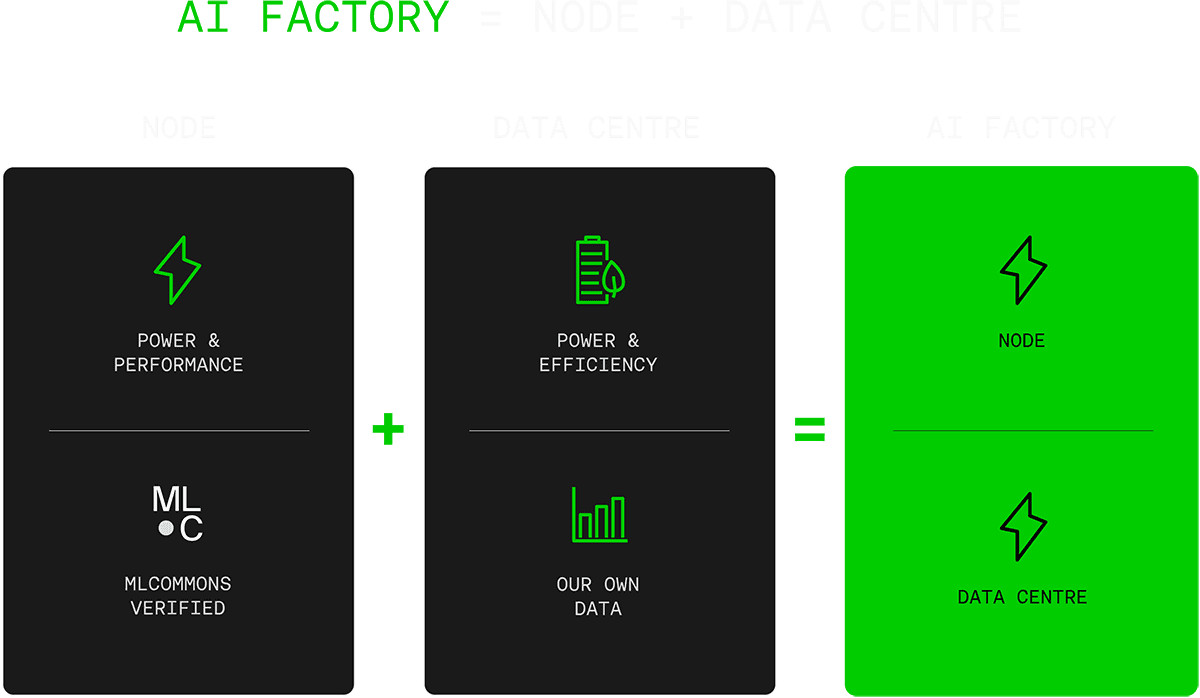
What have we measured?
For each MLPerf® training result, we measured the power consumption of each 'node' or GPU server. This was captured as far upstream as possible - at the level of our immersion-rack power shelves - to accurately assess each node's power consumption. This portion of our MLPerf® submission was reviewed and verified by MLCommons and other members. At this level, our results show approximately 30% better performance compared to H100 SXM-based systems cooled with air, the most common method for building GPU clusters today.
However, capturing power at the node is only part of the story. The host data center plays an incredibly important role in measuring the energy efficiency of an AI Factory. Data center power usage was not within the scope of this round of MLPerf®, but we hope it will be included in future evaluations. Measured by PUE (Power Usage Effectiveness), our data center level provides a (non-MLCommons-verified) estimate of the total power required for the relevant test - end to end.
The benchmark: SMC has cut AI's energy use by over 50%
By combining benchmarked power consumption at the node level with net data center mechanical efficiency, we are proud to reveal - for the first time in the industry - the full power impact of building AI factories. For this MLPerf® Training run, we focus on these types of training workloads. In future releases, we will also benchmark inferencing.
And the results? As we're the first and only submitter of power data for this initial training run, we've provided a calculator on this page to help you compare the efficiency of our Sustainable AI Factor-powered approach versus the legacy way things are done today.